U-M Health Lab: New Analytic Tool Designed to Help Guide Precision Cancer Research and Treatment
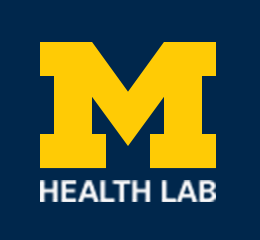
Recent large scale efforts to categorize the molecular data of multiple cancer types has yielded so much information that researchers now have a new question: How to turn all this data into meaningful information that guides cancer research and patient care.
A new analytic tool developed by University of Michigan Rogel Cancer Center researchers combines multiple data sets to help sift the signal from the noise.
“Our idea was to combine three sources of data sets – molecular data from both cancer cell lines and patients and drug profiling data – to understand proper preclinical models that are most representative of these tumors,” says Veerabhadran Baladandayuthapani, Ph.D., professor of biostatistics at the University of Michigan School of Public Health and senior author of a paper published in the Journal of Clinical Oncology Clinical Cancer Informatics that describes this new tool.
The tool, called TransPRECISE, uses data from 7,714 patient samples across 31 cancer types, collected as part of the Cancer Proteome Atlas. This is combined with 640 cancer cell lines from the MD Anderson Cell Lines Project and drug sensitivity data representing 481 drugs from the Genomics of Drug Sensitivity in Cancer model system.
Like Podcasts? Add the Michigan Medicine News Break to your Alexa-enabled device or subscribe for updates on iTunes, Google Play and Stitcher.
“The good thing is this is a very dynamic process. We can have this whole system set up in a computer. As new patients come in or new data comes in, you can keep adding it,” says Rupam Bhattacharrya, M.Stat., a doctoral student and first author on the paper.
The tool builds on an earlier model the team had created, which they called PRECISE. With an eye toward precision medicine, they created a model to look at what changes occur to the molecular structure of individual patients’ individual tumors. TransPRECISE adds in data from cell lines and drug sensitivity, which will be helpful for researchers translating cancer cell biology into drug discovery.
“Now that we have tens of thousands of tumors on these patients we can evaluate what might be the potential therapeutic efficiency of these drugs. The key idea was to develop an analytic tool to do that,” says Baladandayuthapani, who is also director of the Rogel Cancer Center’s cancer data science shared resource.
MORE FROM THE LAB: Subscribe to our weekly newsletter
In the JCO Clinical Cancer Informatics paper, researchers validated the tool by comparing known drug responses and clinical outcomes in patient data. TransPRECISE identified the differences in proteins among individual tumors and accurately tied it back to actual patient outcomes. In addition, they looked at several pathways to predict potential drug targets. This yielded results that mirrored current treatment recommendations or targets being tested in clinical trials, such as ibrutinib for BRCA-positive breast cancer, and lapatinib for colon cancer.
“We have so much data, how do we drill it down to make it more informative so an oncologist can understand? Our work would potentially help oncologists or researchers develop concrete hypotheses based on which mechanism is working, potentially bringing to the top drugs that might warrant more evaluation,” Baladandayuthapani says.
The researchers have made publicly available a comprehensive database and visualization of the findings at https://bayesrx.shinyapps.io/TransPRECISE.
Paper cited: “Personalized Network Modeling of the Pan-Cancer Patient and Cell Line Interactome,” Journal of Clinical Oncology Clinical Cancer Informatics. DOI: 10.1200/CCI.19.00140